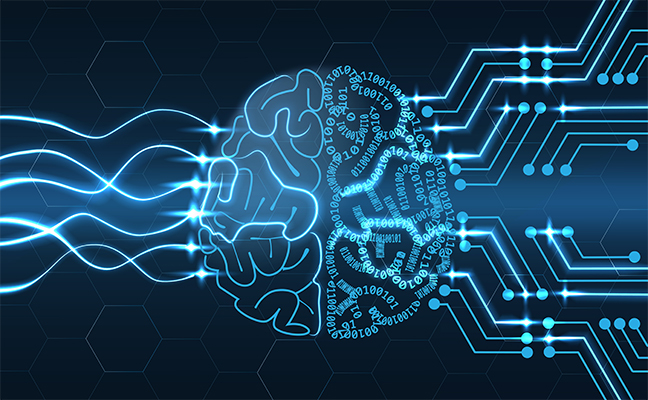
Artificial intelligence (AI) has rapidly become an indispensable part of modern, lean product development. However, there remains a cloud of confusion around what AI is truly capable of, how impactful it can be, and the practical steps teams should take to leverage it effectively. In this post, we’ll clarify some major misconceptions, provide best practices for integrating AI, and showcase why it should be a core priority for any modern product roadmap when used responsibly.
The Rise of AI in Personalizing and Optimizing Digital Experiences
Let’s ground this discussion by highlighting some of the most common and valuable applications of AI that are elevating user experiences and business metrics:
- Personalization: Sophisticated AI algorithms can now tailor individual interfaces and content recommendations to match each customer’s preferences. Services like Netflix and Spotify owe much of their addictive stickiness to this personalization capability.
- Recommendations: Users have come to expect apps and sites to proactively suggest new products, features, or media tailored specifically to their tastes. Beyond entertainment, AI recommendation engines also aid discovery in markets like fintech and e-commerce.
- Predictive Analytics: AI analytic techniques empower managers to detect macro usage trends, forecast business metrics, model likely customer behaviors, and quickly spot anomalies. This is invaluable for tasks like churn reduction, demand planning, and fraud prevention.
- Sentiment Analysis: Understanding subjective emotions and reactions is difficult for computers. But using AI, they can now automatically parse mountains of qualitative feedback from sources like app reviews. This equips teams to track brand sentiment and fine-tune offerings.
- Automated Testing: Performing comprehensive testing across the myriad flows and interfaces in modern digital experiences is extremely challenging. AI techniques like computer vision, natural language processing, and robotic process automation are now being leveraged to automatically navigate apps, enter diverse test data, compare expected vs. actual outcomes, detect visual regressions, and generate detailed test reports without tedious manual effort. By increasing testing thoroughness and velocity, AI test automation helps developers ship higher-quality code faster. Leading technology firms now use AI bots that autonomously explore mobile and web apps to uncover flaws without the need for human testers to write scripts. Though still maturing, such intelligent test agents are a glimpse into the future of automated QA.
As these use cases illustrate, AI should be viewed as an amplifier, not a panacea. When properly integrated with smart processes and talented people, it becomes an incredible lever that unlocks immense latent value.
Addressing Key Myths and Misconceptions
While enthusiasm for AI abounds, inflated expectations combined with a lack of technical knowledge have birthed several misconceptions that deserve clarification:
Myth #1: AI eliminates the need for human insight and oversight.
Reality: AI systems always demand diligent governance. Though increasingly autonomous in surface applications like chatbots, they cannot match humans in areas like abstraction, creativity, ethics, and empathy. Rather than full automation, the most prudent path forward is augmented intelligence and meaningful human + machine symbiosis. It’s important to recognize the steps of a workflow where it makes sense to leverage AI, the steps where humans are best suited to serve today, and an automation roadmap that factors in data gathering and tuning future iterations of the model based on the usage of the early versions.
Myth #2: Off-the-shelf AI solutions will meet all product needs.
Reality: The quality of AI depends greatly on the volume and variety of data it learns from. As a result, pre-built models with generic datasets rarely deliver acceptable performance without extensive adaptation. Customization aligned to your niche use case is thus imperative.
Myth #3: AI techniques can extract insights from any dataset.
Reality: AI algorithms have diverse assumptions and data prerequisites. Proper selection and preprocessing of data is therefore critical – not all datasets are ripe for AI nor useful for the specific business challenge. Creativity is knowing your goal and then finding or creating the appropriate data.
Once these misconceptions are addressed, product teams can shift focus to pragmatic steps for leveraging AI judiciously. Doing so will allow teams to move forward with the best product practices layered with AI to deliver strong results in a low-risk, high-reward manner.
Actionable Best Practices for Integrating AI
While earlier stages of AI adoption focused on chasing technical novelty, maturing organizations now prioritize tangible value creation. We’ll cover some key best practices through this product-centric lens:
Align Potential AI Applications to Business Goals
Don’t let AI be an end unto itself – have a clear vision for how improved personalization, forecasting accuracy, or any other capability translates to driving business outcomes. Choose the best toolset and models based on their impact on revenue, retention, or other concrete business priorities.
Foster Cross-Functional Collaboration with AI Experts
Data scientists specialize in model creation, statistical analysis, and pipeline development. However, product managers and UX designers understand user pain points and commercial outcomes better. Increased collaboration unifies these strengths.
Take an Iterative Approach to AI System Development and Deployment
The first AI algorithm almost never gets it right, so plan from the outset for successive iterations based on real user data. Avoid overengineering complex systems upfront. Start simple, test quickly with a lean prototype, and then expand scope. Remember that feedback from the early stages of the product can be more useful than the team’s instincts in prioritizing the next features.
Focus on Creating Measurable Business Value Over Chasing Technological Novelty
Guard against “shiny new tech” syndrome. The latest deep learning architecture with a flashy whitepaper is irrelevant if it does not demonstrably improve metrics. Measure results ruthlessly and stay aligned to business KPIs, not novelty for its own sake.
Be Obsessively User-Centric
AI presents fantastic opportunities to delight customers by learning from their behavior to serve them better. However, improperly deployed systems can alienate users, manipulate them, or violate privacy bounds. User needs should remain the North Star guiding ethical AI adoption. Customer research, usability testing, and identifying your smallest viable market as a starting point are easier than you think.
Make Ethical Considerations a Priority From Day One
Speaking of ethics, the potentially harmful societal impacts of AI are finally getting their due attention. Consider fairness, interpretability, transparency, bias mitigation and security from the earliest stages when evaluating AI viability in your products.
The Bright Future of AI in Digital Experiences
If those practices are followed diligently, AI integration can provide a formidable competitive edge. Here is a small sample of the incredibly promising AI capabilities emerging that digitally savvy product teams have to look forward to:
- Hybrid AI assistants combining conversational and visual interfaces
- Lifelike computer vision capabilities in augmented reality
- Predictive maintenance analytics minimizing hardware downtime
- Causality-based models elucidating complex scientific phenomena
- Federated learning for privacy-centric personalization
- Lightweight AI deployment on edge devices
With innovation proceeding rapidly across AI subfields, prioritizing a thoughtful integration strategy tailored to your niche now will pay dividends. Be bold yet pragmatic in unlocking the tremendous potential while mitigating the risks. If done properly, artificial intelligence can transition from buzzword hype to become an integral driver of your product roadmap and commercial success.
Get in touch if you’re ready to begin your AI journey or just want to chat about the possibilities.